As artificial intelligence (AI) has become more widely discussed and pursued in business, most professionals have come to learn that AI is concerned with devising computational methods to emulate human intelligence. It is hoped that, eventually, AI can be improved to the point of exceeding human capability as has been depicted in an entertaining fashion in many machine-versus-human competitive games that have been staged in recent years—take for example the matches between chess grandmasters and IBM’s Deep Blue supercomputer.
The bright hope for AI goes beyond winning a chess game, of course. In fact, there is tremendous optimism that AI can solve problems ranging from finding cures for some of the most life-threatening diseases to predicting terrorist threats to sounding the alarm and averting widespread devastation from natural disasters.
Development of AI
AI originated in the 1940s, spurred by the character Tin Man—a metal man without a heart—from The Wizard of Oz.[1] Following this, British polymath Alan Turing significantly advanced the concept of intelligent machines when he published his October 1950 paper, Computing Machinery and Intelligence.[2] Since then, AI has been developed, refined, and continuously assimilated into our lives.
Unknowingly, we use AI in our daily lives; we use it to chart our course to work in the morning, ask virtual assistants for the weather, and search for interesting articles about the applications of artificial intelligence in health care. In fact, the application and use of AI is growing across all industries and markets. The global AI market is expected to grow to over $350 billion by 2028, at a combined annual growth rate of 33.6% between 2021 and 2028.[3]
Machine learning (ML) is one technique used to power AI applications. ML involves training machines to learn from past data, spot patterns, and make predictions that drive increased efficiency and improved decision-making. Eighty-three percent of managers in the U.S. are currently using or planning to use AI in the next few years.[4] As a result, demand for ML engineers and data scientists is increasing because they have the skills and knowledge required to develop ML models. Equally, these heroes of AI and ML are continuously seeking interesting and relevant problems to solve with their skills that have a meaningful impact on society.
Applications in Health Care
Health care presents virtually infinite opportunities for the application of machine learning. For instance, clinicians have vast amounts of patient data available to them but not enough time to review and determine what, if any, of it is relevant to providing improved care to their patient. Additionally, the pressure that the COVID-19 pandemic has placed on medical professionals has accelerated retirements and career changes out of clinical work such that we are facing shortages of clinicians. For patients, who are often battling multiple conditions and unique circumstances, the risk of providing incorrect treatments or drugs is high, which has stressed the importance of developing personalized health care solutions that address individual needs. Frankly, we have only begun to scratch the surface of health-related questions to be answered.
As Alan Turing said long ago, We can only see a short distance ahead, but we can see plenty there that needs to be done. Thankfully, an increasing number of teams are tackling these issues; we highlight below two young, innovative companies making remarkable progress.
SkinIO
SkinIO was developed by Kyoko Crawford and Dr. J.C. Lapiere with the goal of making skin cancer detection more efficient and accessible. The company’s core intellectual property applies AI and ML to identify skin lesions of concern and make the screening process more efficient. With 350 million residents in the U.S. and fewer than 10,000 dermatologists trained to screen for skin cancer, it is mathematically impossible to scale in-person screenings and reach everyone in need, so SkinIO has developed a solution to this challenge.
The full workflow involves a patient doing a virtual full-body skin exam at home via an app or onsite at the workplace. The images are then securely and confidentially uploaded to a server, automated AI-powered mapping of moles on the skin is performed for the clinician to review, and triage is done to follow-up care as necessary. SkinIO’s total body photography, paired with AI-powered image analysis, helps patients map their entire skin surface and figure out what matters, outside of an inaccessible dermatologist’s office.
AI supports every aspect of the process. It begins by guiding the patient to take good quality images of their skin and provides them with real-time feedback via a software downloaded to a person’s phone (an app). Following this, the AI detects, sizes, and categorizes the moles. Finally, it enables outlier detection, or the “ugly duckling sign,” to see whether the identified moles differ from the rest and need to be marked for closer review or follow-up.
SkinIO’s AI technology functions as the dermatologist’s second set of eyes, helping them triage cases and save more lives than otherwise possible. Outside of the medical office, SkinIO provides a transformed experience for patients who can screen themselves for skin cancer from the comfort of their home. It also means the millions of patients diagnosed with skin cancer can bypass long waiting times—32 days on average and 200-plus days in some markets[5]—to see one of the only three dermatologists for every 100,000 people in the United States.
The muscle behind SkinIO’s AI is its team of engineers, app developers, and data scientists who balance the efficiency and accuracy of the AI algorithm to get the optimal clinical and cost outcome.
The idea of accessibility is critically important to the team at SkinIO. Through its app, the company has made screenings accessible to a wider population than ever before. However, the SkinIO team does not want to stop with just skin cancer. They believe the concept and technology can have profound impacts when applied to other conditions and in making progress on broader societal issues of health disparity. Given the uneven distribution of health care resources across the country, by creating intelligent, virtually accessible apps, critical preventative measures can be made accessible to everyone. SkinIO intends to continue to utilize the growing computational power on our phones to save lives.
Nuna
Nuna’s Meaningful Matches product is another example where ML is being deployed to change outcomes in the health care sector. Founded by Jini Kim and David Chen, Nuna’s products and services aim to guide patients through a complex health care system and improve outcomes. A key product in its suite, Meaningful Matches uses advanced data science to match members to primary care providers (PCP) who are available, accessible, and high quality for them.
Choosing one’s doctor can be difficult. A patient needs to consider what they need in terms of convenience, health requirements, and other personal preferences. As we all know, it can be hard to find a person to cut our hair, let alone find a doctor that could be asked to perform services that could save our life. Nuna’s Meaningful Matches product simplifies this task by taking the gold mine of member and provider data available to health plans and finding the most appropriate match based on criteria that matters the most, statistically and personally.
The multiple ML models that underpin the product are trained offline using the health plan’s historical claims data and generate predictions for the providers on four key criteria: cost, quality, retention, and compliance. In real-time, the application programming interface (API) ingests members who need a PCP and their preferences, narrows down PCP choices following the health plan’s business rules, and creates an ideal match based on cohorts, risk scores, geographic accessibility, and availability. Responsiveness and relevance are critical at a time when there is a torrent of information available to patients and they need a simple and personalized way to find the right health care solution for them. Meaningful Matches tailors health care provider recommendations and channels them to users through auto-assignment, search functions on health plan sites, and call centers—all to improve accessibility and engagement.
To the Nuna team, it is paramount that the results from the models are fair (free of bias) and easily understandable to ensure users can trust in the process. To that end, the team at Nuna is spending significant effort on the objectives of fairness and transparency. The Nuna team incorporates business rules that align health plans’ broader goals around cost management with quality, and provider and customer experience. Given the wealth of provider and patient information available to Nuna, the team is also acutely conscious of the potential to address accessibility issues and health disparities. As an example, Nuna is currently exploring using socioeconomic and demographic data to account for access issues, as well as provider quality bias.
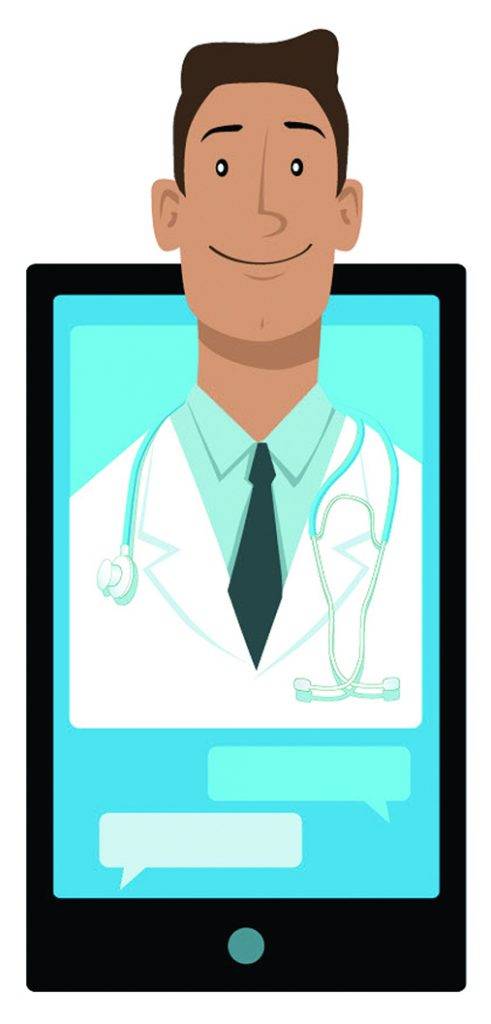
To combat the potential risk of unintentionally introducing bias when using ML, Nuna stratifies its health plan data and runs consistent tests to ensure that patients are not negatively impacted. For example, in order to add a new feature to a model such as socioeconomic data, Nuna compares the PCP matches generated using variations of this feature to a control group. If it were to be found that members with lower socioeconomic status receive lower-scoring providers, Nuna would not incorporate that feature into the model.
With so much data being analyzed and synthesized by intelligent algorithms, the use of ML also introduces the risk of the outcome being a “black box” to the end customer. Building the patient’s trust is a top priority and Nuna invests heavily in understanding and explaining the reasons for patient matches.
Like SkinIO, the Nuna team powering the ML solution consists of product managers, data scientists, engineers, and actuaries, who work with the product and other teams to ensure a holistic solution. The team at Nuna is taking aim at the bigger picture of improved health care and ML helps solve a significant part of the problem by driving efficiencies and tailoring the user experience.
While the team has driven significant innovation that has already greatly benefitted the health care community, they still have many blue-sky aspirations they are driving toward that they believe would be game-changing. Unlimited storage capacity, without impacting the speed of computing, is the Nuna team’s dream. With this, the team could expand its reach and leverage even more detailed high-frequency data—whether it be clinical or community information—that could be used to not only improve the user journey and optimize patient decisions but also impact broader societal outcomes such as reducing health disparities.
The Role of Venture Capital in AI
Both SkinIO and Nuna are companies funded by private capital. Work done in a firm funded by venture money has—by necessity—a rhyme that fits well with learning through AI. To survive, venture firms must have a vision that is appealing to customers and (quickly) proven by results. The pressure to produce results—where there were no results previously—sets up a repeating cycle of talking to customers, identifying opportunities, identifying new sources of information, trial, failed theory, and learning. Successful AI speeds up the identification of new opportunities and more quickly confirms or disproves theories. Faster learning through AI is likely to be one of the drivers of venture capital for the foreseeable future.
Impressive AI work is also being done by established companies. For example, Google’s 1,000-person DeepMind division recently released high-quality predictions for the shape of every single protein in the human body—a discovery that is compared to the mapping of the human genome. As society seeks solutions such as cures for diseases, researchers will benefit from information about the structure of proteins. It can be noted that DeepMind was acquired by Google as a 75-person company in 2014.
Conclusion
We are optimistic about AI and ML in health care. The current and emerging innovators in health care that leverage AI and ML techniques to solve problems are focused not only on solving the problems of today, but also on what the possibilities are for tomorrow. The rapid pace of clinical innovation is testament to this mindset.
Additionally, these bright minds are also wrestling every day with the challenges and responsibilities of utilizing data and such powerful techniques. As they say, with great power comes great responsibility, and it is one that is not being taken lightly as we progress to a future that is increasingly data-driven.
Surbhi Misra, Dave Nelson, and Keith Passwater operate as part of PascoAdvisers, a health care risk strategy and advisory firm.
References
[1] Anyoha, R. (2017), “The History of Artificial Intelligence”, Special Edition on Artificial Intelligence, August 28, 2017 [Blog]. Available at https://sitn.hms.harvard.edu/flash/2017/history-artificial-intelligence/ (Accessed January 4, 2022). [2] See Mind, Volume LIX, Issue 236, October 1950, Pages 433-460, https://doi.org/10.1093/mind/LIX.236.433 [3] 2021, “Artificial Intelligence (AI) Market to Hit USD 360.36 Billion by 2028; Surging Innovation in Artificial Internet of Things (AIoT) to Augment Growth”, Fortune Business Insights, September 16. Available at https://www.globenewswire.com/news-release/2021/09/16/2298078/0/en/Artificial-Intelligence-AI-Market-to-Hit-USD-360-36-Billion-by-2028-Surging-Innovation-in-Artificial-Internet-of-Things-AIoT-to-Augment-Growth-Fortune-Business-Insights.html (Accessed January 4, 2022). [4] “The Future of Work: Adapting to technological change”, Robert Half Talent Solutions, 2019. Available at https://www.roberthalf.com/research-and-insights/workplace-research/the-future-of-work (Accessed January 4, 2022). [5] Borve, A. (2019), “Dermatologist Near Me? Not Likely…”, First Derm, December 3, 2019 [Blog]. Available at https://www.firstderm.com/dermatologist-near-me/ (Accessed January 5, 2022).