How persuasive chatbots might be used in insurance
By Srivathsan Karanai Margan
Chatbots are software programs that were originally designed to mimic human conversations. Over the years, technological growth in artificial intelligence (AI) and natural language processing (NLP) has resulted in tremendous progress in the capabilities of this humanized interface. Consequent to the success, companies are starting to deploy chatbots as an additional customer engagement channel to have a dialogue with users, answer their queries, and perform actions based on the intent.
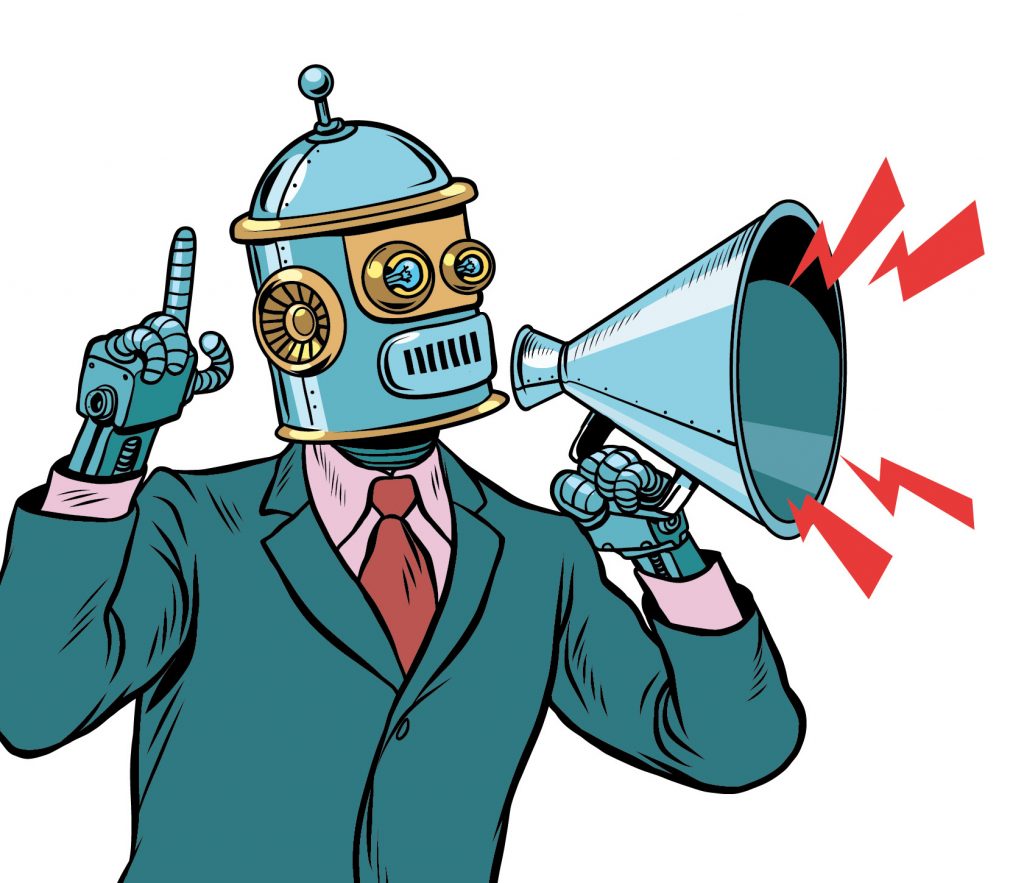
As chatbots mature to play the role of digital front-office executives, companies need to replicate the elements of human-to-human interaction as much as possible. This article explores how chatbots could be designed to persuasively converse with a user to achieve a behavioral change, how persuasive chatbots could support insurance operations, and future possibilities as both conversational and supporting technologies continue to evolve.
The New Engagement Channel
Creating software programs that could mimic human conversation remained a technological challenge for several decades. Due to the progress in AI and NLP, this gold standard to evaluate AI has been reasonably achieved. Today, chatbots can imitate humans and converse with users in natural language. Companies are starting to implement chatbots as a customer engagement channel, thus reflecting a potential shift in the way companies interact with their customers, exchange data, and provide services.
The chatbots fall under two broader typologies: rule-based and AI-based. The rule-based chatbots, also known as decision-tree-based, are commonly deployed by companies for customer-facing business functions. These are less complex, faster to build, and easy to maintain. Rule-based chatbots are based on manually preset “if-this-then-that” conditional rules. They depend on keywords from controlled inputs of users to fetch and push predefined answers or direct customers to appropriate resources. These chatbots exhibit limited capabilities and are harder to scale.
On the other hand, AI-based chatbots, also known as corpus-based, are characterized by their hierarchical structure of language comprehension and logical reasoning. These chatbots understand the context of unstructured user inputs, even when they have typos and grammatical errors. These chatbots mine through a corpus of conversations that is relevant to the dialogue context to produce a response. They achieve this in two ways. First is by understanding the dialogue context and retrieving information from a corpus by searching for matching patterns (retrieval-based chatbots). The second is by generating the response using pretrained language models (generative chatbots).
Retrieval-based chatbots are built on NLP to parse the input, detect intent, understand the context and sentiment, match keywords with existing patterns, and respond in an appropriate format. These bots give users a free hand to input their queries in natural language. Generative chatbots are trained on large general language corpora for grammar, syntax, dialogue reasoning, decision-making, and language generation. Generative chatbots are currently in the early stages of research and are used to build systems for having open-ended conversations that do not have any specific goal or task to perform. These must be trained on large domain corpora to make them task-oriented dialogue systems that possess explicit or implicit goals to support specific tasks.
The rule-based bots are stateless, which means they have no memory of the previous dialogues and focus only on responding to the input that has just arrived. However, the AI-based bots could be semi-stateful or stateful, which means they could possess varying levels of memory and knowledge about the previous dialogues and conversation history. In the recent past, a hybrid of rule-based and retrieval-based has evolved and seen more business acceptance. These chatbots use NLP to extract intents from unstructured user inputs, understand them, and then follow a decision tree to guide the conversation flow.
The Need for Persuasive Chatbots
The increased drive by companies to digitalize and offer touch-free services is increasing the importance of chatbots in customer service. From simple rule-based first-generation chatbots that merely answered to a finite list of frequently asked questions (FAQs), the current generation of the hybrid-chatbots answer personalized queries, in addition to handling service requests for transactions.
As regards business queries and transactions, certain tasks—such as buying a new product or investing more money—are perceived to be positive by a company, and a customer is encouraged to finish them. Against these, a function like a customer wanting to cancel a contract is perceived to be negative and not preferred by a company. In both situations, the customers would have their reasons to do or not to do an activity. While taking any decision, people are supposed to consider all the viable options and alternatives and weigh the evidence to pick a rational choice. However, the paradox is people have cognitive and emotional biases that limit their ability to think objectively. When they face uncertainties, people are not able to systematically describe the problems, record all the necessary data relevant to the situation, or synthesize information to create rules for making decisions. With a constrained vision that is tunneled due to short-term compulsions, they depend on subjective and less ideal paths of reasoning and end up making irrational choices.
In a human-to-human engagement model, an intermediary or representative from a company could interact with the customer during the process of decision-making to understand the situation, present relevant facts, point out ignored data points, discuss with empathy, and nudge them toward or against a decision. Due to the absence of such engagement in a digitalized environment, the customer’s choice to perform a negative task or ignore to perform a positive task could potentially pass unchecked. This shifts the onus for intervention and persuasion to intelligent systems. Chatbots as the fallback conversational channel in the digital environment must evolve as a surrogate for humans and engage with users to persuade them toward a targeted behavioral status.
Persuasion With Chatbots
Persuasion is a goal-directed and value-laden activity that is defined by APA Dictionary of Psychology as “an active attempt by one person to change another person’s attitudes, beliefs, or emotions associated with some issue, person, concept or object.” The process of persuasion comprises steps such as assessing the situation and needs of the persuadee, building rapport, focusing on the benefits, listening to and addressing the concerns, accepting the limitations, finding a common ground of acceptance, and clarifying the final terms. Humans can do this with an effective blend of verbal and nonverbal components of communication. The nonverbal components such as vocal tone, facial expressions, head movement, eye contact, hand gestures, body posture, and physical distance play a very powerful role to amplify the appeal of the verbal component. Without having any real nonverbal component, the persuasive dialogues that chatbots have should depend only on the textual message and some nonverbal proxies to synthesize the appeal. Notwithstanding this limitation, having a decent conversation will be more effective than the passive nudge with persuasive design components.
Generative chatbots are yet to master both domain knowledge and common sense. Consequently, they are risky to deploy for any serious commercial activity. Only pure rule-based and hybrid chatbots with limited ability to handle all the nuances of persuasion dominate the current industrial implementations. However, with a proper conception and design, these chatbots could be still built to persuade. The art of persuasion is primarily categorized in two types—hard and soft. While hard persuasion involves using authoritative power, evidence, reason, and argument, soft persuasion involves emotional and imaginative appeal. Chatbots could follow the path of smart persuasion: an ideal combination of both soft and hard.
The design of a persuasive chatbot can begin with the identification of the persuadable events from the list of tasks a chatbot is deployed to perform. The next important decision depends on whether it should be a reactive or proactive. Reactive persuasion will be in response to a user-driven action seeking some information or proceeding to perform a persuadable trigger event. Depending on whether the event is positive or negative, the response could be to encourage or discourage. On the other hand, proactive persuasion will be chatbot-driven and auto-triggered. It will happen when a user accesses the chatbot for some other purpose and after completing the task, the persuasive dialogue for a different event gets triggered. A simple example of proactive persuasion is to influence to cross-sell a new product after completing the task for which the user had logged in.
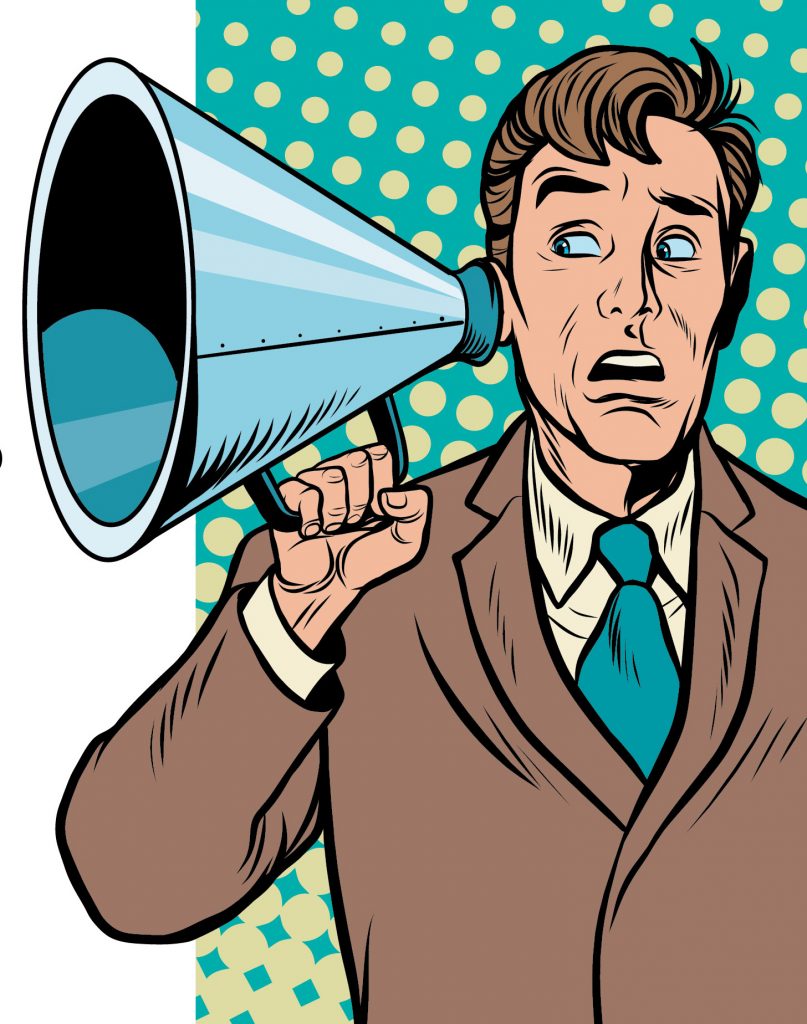
It will be a prerequisite for companies to create a consolidated view of the customer and integrate it with the chatbot to handle complex and personalized proactive persuasion. On this data, intelligence will have to be built to identify the persuadable events from data, prioritize them for their importance and urgency, identify the relevance, time the exact moment and method to introduce the context in an ongoing conversation, and orchestrate the flow of the conversation.
Leveraging Richard Braddock’s Communication Model
The communication model proposed by Richard Braddock as an extension of “the Lasswell Formula” could be considered as a theoretical framework to design the attributes of a persuasive chatbot. The model describes an act of communication by defining “who says (communicator), what (message), to whom (audience), under what circumstances (situation), through what medium (channel), for what purpose (motive, objective), with what effect (effect, result)”.
Each element in this framework could be further augmented by incorporating the sub-elements suggested in the following models for communication and persuasion:
Who Says
“Who says” refers to the communicator, which is a chatbot. The status in the SCARF model relating to how you see yourself and how others see could symbolize the “who says” element. The chatbot inherits some of the trust and credibility from the company it represents. However, it will be able to derive real authority only from its ability to correctly understand the user inputs, perform the tasks it promises that it would, and be consistent. The chatbot could gain the authority of intelligence if it is based on an elaborate decision tree that considers several scenarios, arguments, and counterarguments for each persuadable event. The “liking” for a chatbot could be created by giving it a human name and face or identifying it as a bot, and its ability to converse in a human-like way. This could be further amplified with transparency, relatedness, and fairness.
The “who says” element could become increasingly powerful as chatbots begin to remember details of previous dialogues and conversations, acquire personality types (Big Five personality traits or Myers-Briggs type indicator) to converse with an attitude, become culturally sensitive, and use realistic virtual avatars to communicate nonverbally and empathetically.
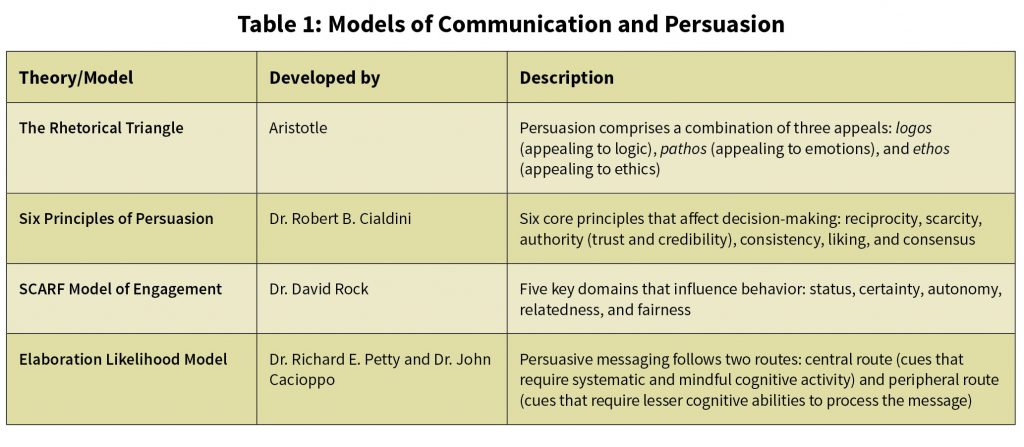
What
“What, refers to the message or content that is being conveyed. This encompasses the overall design of the conversation flow for persuasion, the decision tree of arguments and counterarguments, message framing methods, and variances for the elaboration routes. The dialogues and artifacts exhibited in the conversation should be validated for their logos, pathos, and ethos elements. Though the personal experience of the self is a powerful influencer, a chatbot is disadvantaged to claim any experience. This shortcoming could be overcome with consensus and relatedness by leveraging collaborative filters and citing examples. Any suggestion, data, or calculation shown as a part of the chat should be personalized and contain verifiable certainty. While persuading, the user should be provided with choices so that their autonomy to decide is protected. If a user persists to proceed with a certain decision, the chatbot should not prevent the user from doing it.
To Whom
“To whom” refers to the receiver, which is the user of a chatbot. The users could be from different demographical cohorts and subscribed to different products of the company. These details could be pulled from the core systems of a company to converse with the user sensitively. To address the user by their name is an easy way to establish a connection. However, to have an effective conversation with users, knowledge about their personality type, mood and emotion, and transactional ego states will be needed. These psychographic details will not be available within the existing database of any company. It may be possible in the future for AI-based chatbots to discern these details from the word choices in the current and past chat history of the user. For now, the hybrid chatbots will have to find a workaround by asking relevant questions to assess some of these values to direct the flow of the conversation.

Under What Circumstances
“Under what circumstances, refers to the situation and reason regarding why the user has connected to the chatbot. The persuasion flow should be sensitive to the gravity of the situation to decide whether it is fit for persuasion. For example, it will not be appropriate to persuade for a new product purchase when the user is bereaved. The initial dialogues should first orient toward procuring more information about the circumstances of the customer before stepping up the act of persuasion. To persuade proactively, the appropriate time to intervene for persuasion will be very important for success. The most effective rule of thumb is to leverage the reciprocity principle by intervening after the successful completion of the task.
Through What Medium
The “medium” refers to the strategies, approaches, methods, and techniques used as a part of the chat. In addition to text, images, interactive graphs, infographics, videos, and calculation tools could be presented. As the cognitive capabilities of the users are not recorded by companies, it is difficult to decide upfront whether the central or peripheral route will be effective with a user. To overcome this, the chatbot could ask leading questions as a part of the chat narrative that could help to select appropriate communication cues.
For What Purpose
The purpose refers to the basic reason for the act of persuasion. Persuasion is not coercion. It should be done only with all fairness and benevolent intention focused on the short- and long-term benefit of the customers. The chatbot should be transparent to disclose the business purpose of the activity, benefit, or detriment a targeted behavior could result in, and why a specific action plan is advised. Other than the morally scrutable and overt motives, there should not be any covert intent for the behavior steering.
With What Effect
“With what effect” refers to the effect analysis. In the chatbot context, this can be considered analyzing the effectiveness of the act of persuasion. To assess the immediate impact of persuasion, chat histories could be analyzed to discern user behavior against chat dialogues. This will help to improve the effectiveness of decision paths through continuous learning. The short-term impact could be assessed by scrutinizing the enterprise systems to check if the user has taken any action regarding the persuaded event through any other channel.

Persuasive chatbots in insurance
Individuals have a different kind of relationship with insurance than what they have with any other product or service. Though being the most effective risk mitigation tool, it still requires a hard push from insurers and regulators to make people purchase. The thought of insurance could evoke every other emotion except joy in an individual. The main reason for this is that insurance is a futuristic promise that assures compensation when a covered risk event happens. This operates exactly opposite to the strong impulse of scarcity and immediacy bias.
As in any other industry, the persuadable events in insurance could be based on reactive or proactive triggers to encourage positive or discourage negative events. Depending on the intelligence ingrained in the back-end systems and the extent customer data is consolidated, the proactive persuasion events could be personalized to a customer and not just limited to generalized promotion of a new product or program. It could be performed for other persuadable events of the same policy for which the chat is in progress or expand to include policy events from other policies of the customer.
An indicative list of the persuadable events in an insurance policy could be categorized as given in Table 2.
For proactive persuasion, a comprehensive mapping matrix of the different persuadable events against the context of the conversation can be created and referred to. Improper persuasion matches may lead to undesired consequences. Persuasion events like contradicting policy events such as an appeal for new product sales during a conversation on reporting a loss, or persuading for more than one policy event in the same conversation such as appeal against surrender in one policy and appeal for policy renewal in another policy will have to be avoided. Wherever possible, a persuasion appeal should be made along with curated alternatives for the customer to select from. For example, raising a loan could be positioned as a better option for a customer who contemplates surrender of a policy contract for its cash value.
Insurers are currently adopting a very cautious approach in extending the servicing capabilities of chatbots. The chatbots are deployed to perform very limited functions such as answering FAQ, handling policy queries, updating policy details, generation of statements, and submission of claims for smaller values. The ability to perform either a positive or negative transaction is still very much restricted. However, it is noteworthy that these chatbots still answer queries on how to perform these transactions. Insurers can begin with conceptualizing and experimenting with persuasive chatbots to appeal when a customer queries about a business event. While proactive persuasion could be initiated for events such as new product launches, participating in connected insurance programs, and any other high-priority policy event, reactive persuasion could be done when a customer asks about the procedures for transferring their policy or cash surrender value of their policy. A persuasive interaction of this nature, which analyzes the pros and cons of an action and suggests alternatives, could be extremely effective when the customer is in the pre-contemplation, contemplation, or preparation stage of the transtheoretical model.
The Future of Conversational Bots
The research in conversational AI is continuously progressing. Considering the experiments and research in conversational AI, we can expect future chatbots to have the ability to empathize, and discern the personality type, mood, emotions, and mindset of the user. In addition to the highly versatile conversational capabilities, these bots could adapt a suitable personality type and respond from the appropriate mindset, avoiding crossed communication transactions. The data scarcity to train generative chatbots to perform domain-oriented tasks could be overcome, thus turning them commercially acceptable. As the back-end systems feed chatbots with deeper insights derived from the data deluge, any interaction could get hyper-personalized. The advancements in synthesized speech could enrich the conversational systems with nonverbal communication capabilities, thereby elevating the pitch for the art of persuasion to match the standards of a skilled human persuader.
SRIVATHSAN KARANAI MARGAN works as an insurance domain consultant at Tata Consultancy Services Limited.
References
Andrews, P., De Boni, M., & Manandhar, S. (2006). Persuasive argumentation in human computer dialogue. AAAI Spring Symposium – Technical Report, SS-06-01.
Aristotle’s Rhetoric. (n.d.). Stanford Encyclopedia of Philosophy. Retrieved June 17, 2021, from https://plato.stanford.edu/entries/aristotle-rhetoric/
BRADDOCK, R. (1958). AN EXTENSION OF THE “LASSWELL FORMULA.” Journal of Communication, 8(2). https://doi.org/10.1111/j.1460-2466.1958.tb01138.x
Brandtzaeg, P. B., & Følstad, A. (2017). Why people use chatbots. Lecture Notes in Computer Science (Including Subseries Lecture Notes in Artificial Intelligence and Lecture Notes in Bioinformatics), 10673 LNCS. https://doi.org/10.1007/978-3-319-70284-1_30
Budzianowski, P., & Vulić, I. (2019). Hello, It’s GPT-2 – How Can I Help You? Towards the Use of Pretrained Language Models for Task-Oriented Dialogue Systems. https://doi.org/10.18653/v1/d19-5602
Cialdini, R. B. (2006). Influence: The psychology of persuasion. In New York, NY, USA: HarperCollins Publishers.
Daniel, J., & Martin, J. H. (2020). Speech and Language Processing Chatbots & Dialogue Systems.
Fogg, B. J. (2003). Persuasive Technology: Using Computers to Change What We Think and Do. In Persuasive Technology: Using Computers to Change What We Think and Do. https://doi.org/10.1016/B978-1-55860-643-2.X5000-8
Gallo, C. (2019, July 15). The Art of Persuasion Hasn’t Changed in 2,000 Years. Harvard Business Review. https://hbr.org/2019/07/the-art-of-persuasion-hasnt-changed-in-2000-years
Gardikiotis, A., & Crano, W. D. (2015). Persuasion Theories. In International Encyclopedia of the Social & Behavioral Sciences: Second Edition. https://doi.org/10.1016/B978-0-08-097086-8.24080-4
LaMorte, W. W. (2018). The Transtheoretical Model (Stages of Change). In Boston University School of Public Health.
Norcross, J. C., Krebs, P. M., & Prochaska, J. O. (2011). Stages of change. Journal of Clinical Psychology, 67(2). https://doi.org/10.1002/jclp.20758
Petty, R. E., & Cacioppo, J. T. (1986). The elaboration likelihood model of persuasion. Advances in Experimental Social Psychology, 19(C). https://doi.org/10.1016/S0065-2601(08)60214-2
Pompian, M. M. (2011). Behavioral finance and wealth management: How to build investment strategies that account for investor biases. In Behavioral Finance and Wealth Management: How to Build Investment Strategies That Account for Investor Biases. https://doi.org/10.1002/9781119202400
Rock, D. (2008). SCARF: A brain-based model for collaborating with and influencing others. NeuroLeadership Journal, 1(1).
Mullainathan, S., & Shafir, E. (2013). Scarcity: why having too little means so much. First edition. New York: Times Books, Henry Holt and Company.
Shi, W., Wang, X., Oh, Y. J., Zhang, J., Sahay, S., & Yu, Z. (2020). Effects of Persuasive Dialogues: Testing Bot Identities and Inquiry Strategies. Conference on Human Factors in Computing Systems – Proceedings. https://doi.org/10.1145/3313831.3376843
Thaler, R. H., & Sunstein, C. R. (2008). Nudge: Improving decisions about health, wealth, and happiness. In Nudge: Improving Decisions about Health, Wealth, and Happiness. https://doi.org/10.1016/s1477-3880(15)30073-6
Wang, X., Shi, W., Kim, R., Oh, Y., Yang, S., Zhang, J., & Yu, Z. (2020). Persuasion for good: Towards a personalized persuasive dialogue system for social good. ACL 2019 – 57th Annual Meeting of the Association for Computational Linguistics, Proceedings of the Conference. https://doi.org/10.18653/v1/p19-1566