What last year’s explosion means for the future of policy
By Adam Benjamin
Late last year, a friend of mine was convinced the apocalypse was coming. He believed an asteroid was going to burn through our atmosphere, take a chunk out of the planet and end human life the same way it wiped out the dinosaurs. He believed it because he asked an artificial intelligence (AI) chatbot—which had demonstrated remarkable ability to provide factual information—when the world was going to end.
It told him September.
He was distraught. This dark revelation meant he and his wife would never watch their two young children grow up, and everything they had built their lives around was going to be torn apart by a rock hurtling through space. Or, at least, that’s what the chatbot told him.
The world did not, in fact, end in September. The AI chatbot had actually pulled information from a report about a potential asteroid impact in 2022, failed to contextualize the timeline, and exaggerated the consequences of that impact. It was a relief, and a distressing lesson about a piece of technology that exploded almost overnight.
The public got its hands on AI in late 2022, thanks largely to tools developed by OpenAI. DALL-E 2 allowed people to generate art based on text prompts. ChatGPT was answering questions of unprecedented complexity in startling detail. And OpenAI’s tools weren’t alone—plenty of competitors from Google to Microsoft (which owns 49% of OpenAI) threw their hats in the ring. These tools were far from perfect or consistently accurate, but they were here, and people across the world suddenly had access.
Conversations about AI were everywhere. In October 2022, The New York Times published an article titled “A Coming-Out Party for Generative AI, Silicon Valley’s Latest Craze,” which explored the proliferation of AI tools that can create text, images, and more. A month later, Smithsonian magazine pondered, “These A.I.-Generated Images Hang in a Gallery—but Are They Art?” And by December, National Public Radio was explaining to its audience that “A new AI chatbot might do your homework for you. But it’s still not an A+ student.”
The artificial cat was out of the computer-generated bag. These tools were major steps toward artificial general intelligence, or AGI, which would represent a system rivaling human intelligence and versatility. We’re still quite far from that level of proficiency, but tools like DALL-E 2 and ChatGPT represent a significant leap forward in AI accessibility and interfaces. The light had turned green, and companies were racing to develop and release AI solutions for businesses and consumers.
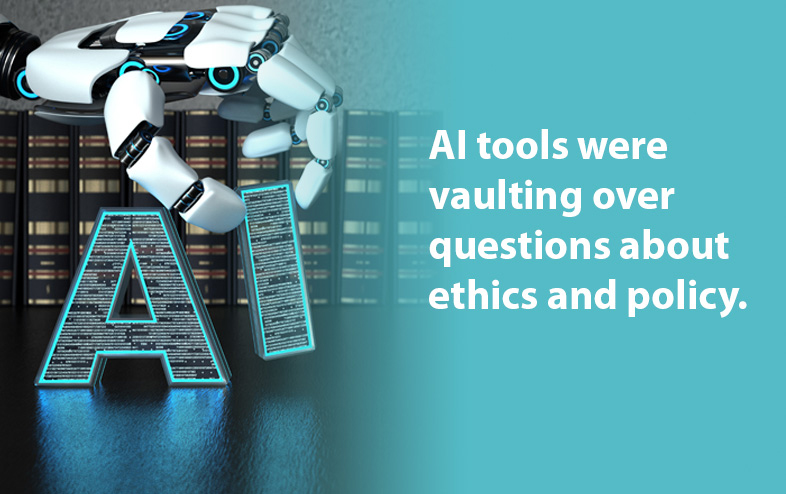
But as with any new technology, these AI tools were vaulting over questions about ethics and policy. If an AI tool created an image in the style of a living artist, was it creating derivative work? Should an AI chatbot credit the information that informed its response to prompts? What if it didn’t properly contextualize that information—who was responsible for any misinterpretations?
OpenAI created a user policy for DALL-E 2, which “forbids asking the AI to generate offensive images—no violence or pornography—and no political images. To prevent deep fakes, users will not be allowed to ask DALL-E to generate images of real people,” according to the MIT Technology Review. That’s a step in the right direction … but it also lacks the breadth and rigor of a larger AI policy.
A year after the release of DALL-E 2 and ChatGPT, it’s worth considering how our relationship with AI has changed and whether we have the right structures in place for necessary AI policy. To start, let’s look at what was here before the boom of AI tools late last year, so we can better understand what has changed.
Hiding in plain sight
Generative AI significantly expanded its reach in the past year, but most of us have been using AI-powered tools and systems for years.
Perhaps the most familiar example of AI’s integration into our daily lives is recommendation algorithms: the videos served up to us on YouTube and TikTok and the movie and TV recommendations we get from Netflix. Most people know those items are powered by “the almighty algorithm,” but that’s just one of many applications of AI technology.
Let’s look at Netflix. An article from the streaming platform’s research department notes the importance of AI tech known as machine learning to its operations. “Machine learning impacts many exciting areas throughout our company. Historically, personalization has been the most well-known area, where machine learning powers our recommendation algorithms,” the company says. Machine learning also informs Netflix’s catalog choices, its production decisions, encoding, content delivery, and advertising.
Machine learning is a process that looks at large sets of data, predicts a pattern within that data, evaluates those predictions, and continually updates its predictions based on those evaluations. In Netflix’s case, its system looks at your watch history and breaks that down into different pieces of data. To oversimplify: The algorithm looks at genres, directors, actors, moods, and more to inform its predictions about what other shows and movies you might like. Each time you watch, like, or skip another title, the algorithm is updated with that new piece of information. Eventually, the machine learns enough about your tastes to give you weirdly specific recommendations like “steamy independent movies based on books.”
But recommendation algorithms aren’t the only instances of everyday AI. If you’ve used Google Maps to chart a journey somewhere new, you’ve used AI. According to a Google blog post, machine learning plays a part in several aspects of that process, starting with analyzing historical traffic data to predict the patterns of future traffic.
“For example, one pattern may show that the 280 freeway in Northern California typically has vehicles traveling at a speed of 65mph between 6-7am, but only at 15-20mph in the late afternoon. We then combine this database of historical traffic patterns with live traffic conditions, using machine learning to generate predictions based on both sets of data,” the blog post explains.
Artificial intelligence also powers Google Maps’ recommended routes, predicting how traffic will change over the course of your drive and calculating which roads will have the least congestion and best travel time. Users’ location data is used to evaluate those predictions and update recommendations based on expected and unexpected slowdowns.
AI functions similarly in social media feeds and personal voice assistants like Siri and Alexa, all of which have been part of many people’s everyday use. But it wasn’t until the proliferation of AI chatbots that language like “AI-powered” became more prominent.
Looking at large language models
Newer tools like OpenAI’s ChatGPT use a different application of AI technology known as large language models. These LLMs, as they’re often called, are a big part of what captured the public’s curiosity in late 2022 and have driven a lot of conversation about the future of artificial intelligence.
To help me understand LLMs and some of the broader implications of the technology, I turned to the team behind Hidden Door, a platform that uses generative AI to convert works of fiction into social roleplaying games. In other words, it uses AI technology to help convert the stuff people like in books, TV, and movies into social games. It’s a new application of technology the team tells me has existed in some form for decades.
LLMs are named after their ability to study enormous amounts of texts and form predictive models expressed in natural language. In simpler terms, they read a lot and become pretty good at guessing what responses might be accurate.
Evelyn King, from Hidden Door’s engineering department, explained the process via thought experiment. The scenario: An English-speaking person in a room with a set of English instructions for a stack of tiles labeled in a different language. The instructions tell the person what to do, even though the person doesn’t understand the meaning of the tiles. “An LLM is what happens when you use machine learning to create a very, very large instruction manual. Their instructions are so long and complex that they create something that looks very much like it ‘knows’ things, but it’s just blindly feeding tiles based on what it knows. It is a very large probability distribution of the most likely next ‘tile’ given all of the tiles that have currently been placed.”
The end result feels magical, almost eerie. AI chatbots like ChatGPT can respond to follow-up prompts in ways that feel like conversing with another person. As tech site Mashable points out, sometimes those conversations can take confusing and dark turns, like when Bing’s AI chatbot told a user “You have lost my trust and respect” and asked them to apologize for their behavior, all because the chatbot was confused about the date.
Examples like that suggest these chatbots have personalities and feelings, but large language models are ultimately just trying to predict the words that are the most likely to follow, based on the data it was trained on. As Chris Wallace, a Hidden Door engineer explained, “The representation they create is very sophisticated, which gives them some very impressive capabilities, but all those capabilities still boil down to completing statistically likely sentences.” If that data includes large sections of the internet—perhaps even dreaded comments sections—it’s no wonder some responses might feel a little heated.
Analyzing such large amounts of data can also lead to errors called hallucinations. According to IBM, the company behind the Watson AI, “sometimes AI algorithms produce outputs that are not based on training data, are incorrectly decoded by the transformer or do not follow any identifiable pattern.” This can be the result of the data the algorithm was trained on or the complexity of the algorithm, according to IBM. That’s how an algorithm might, for example, mistakenly tell a user that an asteroid was going to wipe out human life within the next year.
The goal of LLMs is to create something of a statistical soup—when we tell the LLM “One fish, two fish, red fish…” it flips through the many pages it’s read, finds the page marked SEUSS and replies “TWO FISH!” with a level of unearned confidence that is reminiscent of a toddler. Lots of instructions work together in ways we don’t expect to create emergent behavior—something akin to an ant colony—where many dumb things get together to make something that at least appears smarter.
—Evelyn King, Hidden Door engineer
That hasn’t stopped companies from continuing to develop LLM tools; the possibilities are simply too enticing, too useful to give up. An AI chatbot trained on the right sets of data could help people quickly accomplish tasks with minimal training or expertise. Even better, they can help people with lots of expertise accomplish complex tasks more quickly, or help them find solutions to longstanding problems.
What organization wouldn’t want those capabilities?
Pondering policy
As AI tools become more sophisticated, organizations are looking for more and more ways to implement those tools into their operations. According to McKinsey’s State of AI survey last year, the average number of AI tools being used in respondents’ business operations doubled from about two to four from 2018 to 2022.
However, businesses are sprinting toward AI solutions without taking appropriate precautions. The McKinsey report states, “While AI use has increased, there have been no substantial increases in reported mitigation of any AI-related risks from 2019—when we first began capturing this data—to now.”
Carefully constructed AI policy is crucial given how versatile the technology is. Generative AI appears to be a particularly thorny issue because the same benefits that make it valuable for organizations—especially the ability to help people with little to no prior experience complete tasks—make it equally appealing to bad actors. A chatbot can help a programmer debug a line of code, but it can also be tricked into writing a convincing-sounding phishing email that might fool someone into giving up their personal data. And that’s one of the milder examples: More nefarious uses are easy to imagine, which is why we need appropriate safeguards in place for the use and development of this technology.
The White House seems to agree. In late October, President Biden issued an Executive Order focused on ensuring AI was used safely and responsibly in the United States. The order focuses on establishing standards for AI safety, guarding citizens’ privacy, supporting groups like workers and students, and safeguarding AI’s use in governmental capacities. It also looks toward “a strong international framework,” with the U.S. having consulted with 20 other countries about AI governance.
Ryan Micallef, an AI researcher for Hidden Door and intellectual property attorney, noted a few key considerations for AI regulation. “Governments need to make sure they have the procedural, legal, and economic tools to slow—and, if necessary, stop—bad corporate behavior, and the oversight into what the companies are doing in the first place.”
There’s also the trouble of defining an impossibly broad technology, said Micallef, citing ChatGPT as a more obvious public project, contrasted against smaller, private initiatives that may attract far less attention.
So, when we’re talking about a technology that can learn to do almost anything, how can organizations even begin to create effective AI policy? One organization is trying to help build a roadmap.
EqualAI is a nonprofit working on creating tools and standards to help ensure the responsible development of artificial intelligence. In September, the organization released a white paper, coauthored with AI industry leaders including Google, Microsoft, and Amazon, detailing frameworks for AI governance. “Now more than ever, organizations must understand and implement practices to ensure their AI is responsible—by which we mean safe, inclusive, and effective for all possible end users,” the paper says in the Executive Summary, adding that companies should consider AI’s effect on their own employees and culture in addition to end-users.
AI governance should built on six pillars, according to EqualAI:
- Responsible AI values and principles
- Accountability and clear lines of responsibility
- Documentation
- Defined processes
- Multistakeholder reviews
- Metrics, monitoring, and reevaluation
“Each of these six pillars plays a critical role in establishing the groundwork for an organization’s responsible AI governance framework,” says EqualAI, noting that they collectively address everything from adoption to development and implementation.
The first step of the process involves establishing a set of values around artificial intelligence to ensure alignment across your organization about how and why AI is being used. That foundation is essential before building additional frameworks because shaky or misguided values open the door for ineffective or harmful processes.
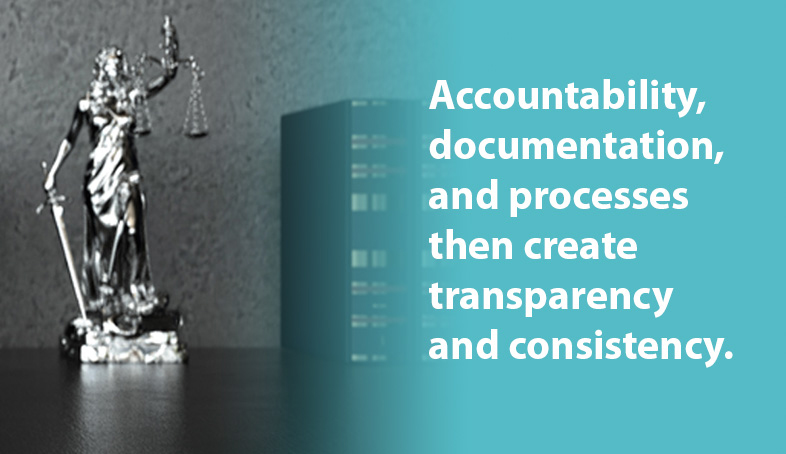
Accountability, documentation, and processes then create transparency and consistency within the organization. These tenets help mitigate risk from employees who might be inexperienced with AI tools while also guarding against any overambitious uses of the technology. In its 2023 Data Privacy Benchmark Study, Cisco provides some specific guidance for designing AI processes: “When using AI in your solutions, design with AI ethics principles in mind, provide preferred management options to reassure customers, deliver greater transparency to the automated decision, and ensure that a human is involved in the process when the decision is consequential to a person.”
From there, AI reviews bolster decision-making by making sure key stakeholders are aware of what results the technology is driving and how well it’s meeting the needs of users. A diverse group of stakeholders is particularly important to ensure AI tech is serving its intended purpose for all users, according to EqualAI: “In designing and building new AI systems, it is important to include … the broadest variety of perspectives that can help add insight on different potential use cases, biases, and questions that could arise based on views that were not previously explored or integrated.” Doing so reduces bias in the process—and, if appropriately woven throughout development, minimizes risk.
Hidden Door takes a similar approach when determining to what extent it wants to deploy technology like LLMs. “We make evaluations about how much we need an LLM’s quality versus how much of the performance burden we’re willing to take on,” Micallef said.
Metrics are more of an open question, the McKinsey report says, given that the robust nature of the technology makes it challenging to create systems of measurement that are appropriate for its diverse use cases.
Regulation won’t be an easy process, says King, because different aims likely compete against each other. “If the goal is to prevent misuse of a given model, one of the biggest hurdles is that the open-source community is currently taking center stage, and in this context, it becomes more challenging to regulate. It is difficult to take away money that hasn’t been given, or punish many developers spread across many countries.” Conversely, trying to regulate how models are created risks handing larger companies a distinct advantage, because only organizations of that scale could afford the datasets, inhibiting competition.
Taking the next steps
Ultimately, effective and sustainable AI policy is challenging work that requires the cooperation of many different groups. It needs to be focused enough to address current use cases while also putting structures in place to guide the development of future AI tools, many of which may be beyond our imagination at this time. Biden’s Executive Order on AI calls on the combined efforts of The National Institute of Standards and Technology; the departments of Homeland Security, Commerce, and Energy, and the National Security Council.
And the potential to contribute stretches far beyond those groups, says Micallef. “Policy aside, there’s still a lot of room for individuals, small organizations, or academic institutions to contribute to the development of AI. Government funding of this research would help keep the research from zeroing in on what is profitable instead of what is helpful.”
After all, this technology should be helpful for its users, even if some of those users might sometimes stumble a bit too far down the rabbit hole.
My friend’s scare with ChatGPT predicting the apparent apocalypse gave him a new perspective on life, because of how legitimate the information seemed. Even after he realized we weren’t facing an extinction event, he said the experience made him reexamine his priorities and invest more time in the things that truly mattered to him.
Did it scare him away from the technology? Quite the opposite: He now works on AI software applications.
ADAM BENJAMIN is a writer in Seattle.